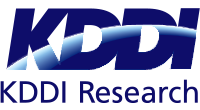
- Advanced Technology Laboratories
Research paper accepted for NeurIPS 2022, a Top AI Conference
November 29, 2022
KDDI Research, Inc.
As a result of the joint work between KDDI Research, Inc. and the University of Illinois at Chicago (hereinafter, UIC), a paper related to continual learning titled “A Theoretical Study on Solving Continual Learning” was accepted on Sept. 15, 2022 for NeurIPS 2022*1, which is a top conference in the field of AI (acceptance rate 25.6%: NeurIPS 2022 announced, presented from Nov. 28 – Dec. 9). This achievement falls into the category of joint research projects with outstanding researchers overseas, which KDDI Research, Inc. has promoted*2.
【Research overview】
Conventional AI tends to forget previously learned classes when learning new data corresponding to a new class. A research group in UIC led by Prof. Bing Liu and KDDI Research, Inc. has been conducting research on how AI can overcome the difficulty of adapting to changes in the environment, e.g., in an animal classification task where new classes like “dog”, “cat”, and so on are added incrementally. The research presented in this paper aimed to achieve “Continual Learning” which is a machine learning methodology to efficiently adapt to previously unseen data while preventing the forgetting of learned classes when training with a new class. With this study, KDDI Research, Inc. aims to develop AI that acquires the ability to adapt to various classes. For instance, this enables the classification of new complicated patterns of network failures while retaining the classification performance on old patterns.
The research theoretically resolves class-incremental learning (hereinafter, CIL)*3, which is known to be an especially difficult task in continual learning. The theory described in the paper decomposes CIL into 1) a change (Out-of-Distribution; OOD) detection problem, i.e., a sub-problem to detect whether newly arrived data are within the data distribution of a new class, and 2) a sub-problem to judge which class among the learned classes the newly arrived data correspond to in the case where the data are not bound to a new class. Under this theoretical decomposition, the experiments described in the paper confirm that CIL performance improves by employing better OOD detection methods. This suggests that OOD detection methods can improve CIL, even though those methods were originally developed outside the context of CIL.
KDDI Research, Inc. aims at establishing “Self-improving AI”, which modifies itself to adapt to frequently changing real-world environments. This paper makes a meaningful contribution in terms of establishing the theoretical foundations of “Self-improving AI”.
【Bibliography】
Gyuhak Kim (UIC), Changnan Xiao (New York University), Tatsuya Konishi, Zixuan Ke (UIC), Bing Liu (UIC), A Theoretical Study on Solving Continual Learning, NeurIPS 2022.
KDDI and KDDI Research formulated “KDDI Accelerate 5.0” (Japanese only), based on a concept for the next-generation society envisioned for 2030, and summarized how it can be accomplished in the “Beyond 5G/6G Whitepaper” in terms of the “Future Image” and “Technologies” required to create an ecosystem that spurs innovation. To develop a new lifestyle, the two companies will continue to invest in R&D on seven different technologies and on an orchestration technology that links them together. The results presented in this study correspond to “AI” as one of the seven technologies.
KDDI Research established the Human-Centered AI Research Institute in April 2022 to promote the research and development of technologies in which humans and AI form a symbiotic relationship and grow together through interaction. In the future, based on the results presented in this paper, KDDI Research, Inc. will continue this line of research to establish “Self-improving AI”, which modifies itself to adapt to frequently changing real-world environments.
(*1)Thirty-sixth Conference on Neural Information Processing Systems
(*2)Launch of Joint Research Projects with Outstanding Researchers Overseas (Press release on October 26, 2020)
(*3)Class-incremental learning is one of the problem settings of continual learning, in which after a model learns a set of classes another new set of classes emerges. For example, if a model learns a dataset containing labels A and B, and then learns another dataset with labels C and D, the model is still required to maintain its performance on the previous data, i.e., samples with labels A and B.
※The information contained in the articles is current at the time of publication.Products, service fees, service content and specifications, contact information, and other details are subject to change without notice.